High Dimensional Data Analysis With Low Dimensional Models: The Ultimate Guide

In the ever-evolving landscape of data science, high dimensional data has emerged as a ubiquitous challenge. With an increasing number of variables and features, traditional data analysis techniques often struggle to provide meaningful insights. Enter the realm of low dimensional models, a powerful toolset that empowers data scientists to navigate the complexities of high dimensional data and extract actionable knowledge.
5 out of 5
Language | : | English |
File size | : | 25795 KB |
Print length | : | 440 pages |
Screen Reader | : | Supported |
The Curse of High Dimensionality
The curse of high dimensionality refers to the exponential increase in the volume of data as the number of dimensions grows. This poses significant challenges for data analysis, including:
- Increased noise and redundancy: High dimensional data often contains a significant amount of redundant and irrelevant information, making it difficult to identify meaningful patterns.
- Computational complexity: Traditional algorithms for data analysis become computationally expensive as the dimensionality increases, making them impractical for large datasets.
- Difficulty in data visualization: Visualizing high dimensional data can be challenging, making it difficult to gain intuitive insights.
The Power of Low Dimensional Models
Low dimensional models offer a solution to the curse of high dimensionality by reducing the number of features while preserving the essential information contained in the original data. This dimensionality reduction process not only enhances computational efficiency but also improves data visualization and facilitates the identification of meaningful patterns.
There are numerous types of low dimensional models, each with its own strengths and applications. Some of the most popular methods include:
- Principal component analysis (PCA): PCA is a linear transformation that identifies the directions of maximum variance in the data, allowing for the projection of the data into a lower-dimensional subspace.
- Multidimensional scaling (MDS): MDS is a non-linear technique that preserves the pairwise distances between data points, making it suitable for visualizing high dimensional data.
- t-distributed stochastic neighbor embedding (t-SNE): t-SNE is a non-linear technique that is particularly effective for visualizing high dimensional data with complex structures.
Applications of Low Dimensional Models
Low dimensional models have a wide range of applications in data science, including:
- Data visualization: Low dimensional models can be used to visualize high dimensional data in a way that is both intuitive and informative.
- Feature selection: Low dimensional models can help identify the most important features in a dataset, aiding in the development of more efficient and accurate machine learning models.
- Clustering: Low dimensional models can be used to group similar data points together, facilitating the identification of patterns and relationships.
- Anomaly detection: Low dimensional models can be used to detect anomalous data points that deviate from the normal distribution.
High dimensional data analysis with low dimensional models is a powerful approach that empowers data scientists to overcome the challenges of high dimensionality and extract meaningful insights. By reducing the dimensionality of the data, low dimensional models enhance computational efficiency, improve data visualization, and facilitate the identification of meaningful patterns. Whether you are a seasoned data scientist or just starting your journey into the world of high dimensional data, this comprehensive guide has provided you with the foundational knowledge and techniques to navigate this challenging landscape confidently.
As the volume and complexity of data continue to grow, low dimensional models will play an increasingly important role in data science. Embrace the power of these techniques to unlock the hidden insights within your high dimensional data and drive informed decision-making.
5 out of 5
Language | : | English |
File size | : | 25795 KB |
Print length | : | 440 pages |
Screen Reader | : | Supported |
Do you want to contribute by writing guest posts on this blog?
Please contact us and send us a resume of previous articles that you have written.
Book
Novel
Page
Chapter
Text
Story
Genre
Reader
Library
Paperback
E-book
Magazine
Newspaper
Paragraph
Sentence
Bookmark
Shelf
Glossary
Bibliography
Foreword
Preface
Synopsis
Annotation
Footnote
Manuscript
Scroll
Codex
Tome
Bestseller
Classics
Library card
Narrative
Biography
Autobiography
Memoir
Reference
Encyclopedia
Trevion Burns
Juliet Marillier
Kat Falls
Nigel C Winter
John W Moffat
Julian Bishop
Jon Henderson
Josh Spencer
Tarik Abdel Monem
Joseph Daniels
Stephen Tvedten
Taylor Kolste
Joshua Zitting
Juan Marbarro
Julian Clary
Kirsten Akens
Jose Mauricio Gaona
Joseph Chaikin
Peter De Cosemo
Michael Bauche
Light bulbAdvertise smarter! Our strategic ad space ensures maximum exposure. Reserve your spot today!


- Dwight BlairFollow ·7.9k
- Nathaniel HawthorneFollow ·6.1k
- Al FosterFollow ·19.2k
- Tom HayesFollow ·15.4k
- Howard PowellFollow ·18.6k
- Felix CarterFollow ·12.9k
- Eli BrooksFollow ·18.3k
- Vernon BlairFollow ·14.8k
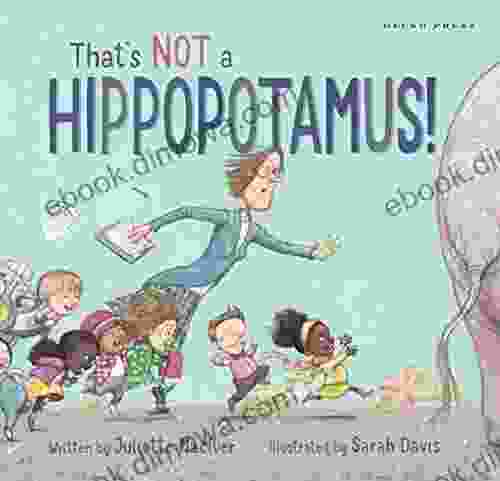

Unleash the Magic Within: "That's Not a Hippopotamus,...
Step into a Realm Where...
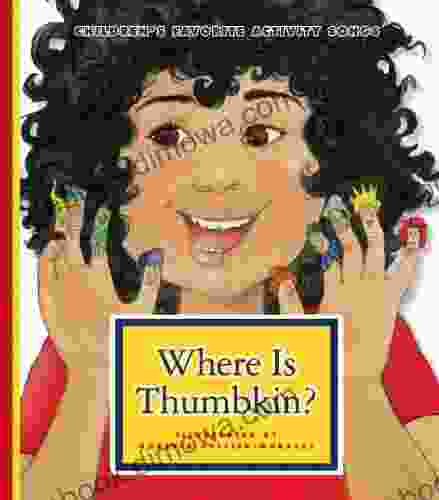

Where Is Thumbkin? A Journey Through Beloved Children's...
In the realm of...
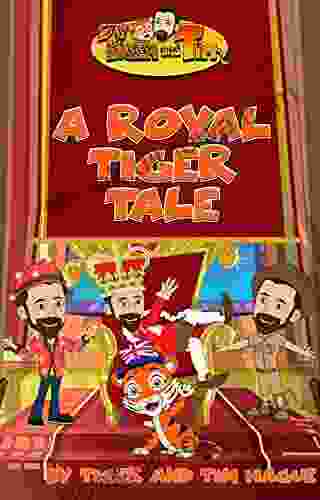

Witness the Unforgettable Journey of "Royal Tiger Tale":...
: Embark on an extraordinary literary...
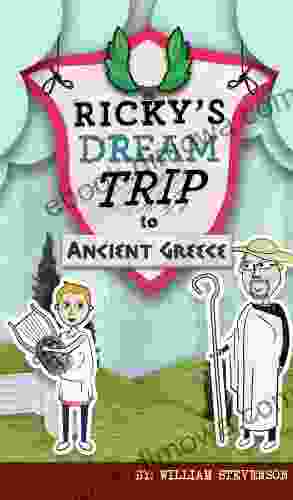

Ricky's Dream Trip to Ancient Greece: An Unforgettable...
Embark on an Epic Journey Get ready...
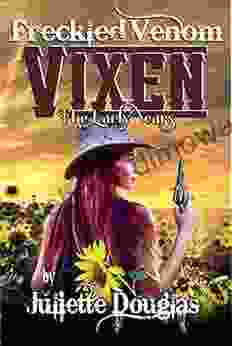

Unleash the Freckled Venom: Embark on an Enchanting...
Dive into the Extraordinary...
5 out of 5
Language | : | English |
File size | : | 25795 KB |
Print length | : | 440 pages |
Screen Reader | : | Supported |